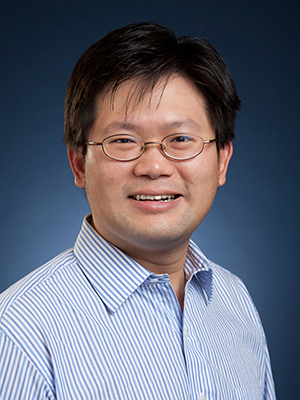
| Stefanus
密歇根大学商学院副教授
|

|
【主讲】Stefanus,密歇根大学商学院助理教授
【时间】2019年6月12日(周三)下午 2:30
Stefanus Jasin is an Associate Professor of Technology and Operations at the Ross School of Business, University of Michigan, Ann Arbor. He received his bachelor degree from UC Berkeley and masters and PhD degrees from Stanford University. Stefanus' main research interest is in developing effective and efficient approximate algorithms for tackling complex and large-scale business analytics problems using tools from statistics, machine learning, computer science, and engineering optimization. He has done works on a variety of topics including dynamic pricing and revenue management, on-demand business analytics, online learning and optimization, and retail logistics and web analytics. He currently serves at the editorial board of Management Science, Production and Operations Management (POM), and Naval Research Logistics (NRL).
【Speaker】 Stefanus, Associate Professor of Technology and Operations at the Ross School of Business, University of Michigan, Ann Arbor.
【Topic】Real-time Dynamic Pricing
【Time】Wednesday, June 12, 2019, 14:30
【Venue】Room 453, Weilun Building, Tsinghua SEM
【Organizer】Department of Management Science and Engineering
【Abstract】 Pricing is without doubt one of the most versatile tool that a seller can use to manage his revenue (or other performance measures). The last two decades have witnessed an explosion of interest in pricing research, most notably because firms nowadays are becoming more aware of its power. And yet, despite its long history, pricing remains a very challenging task, both practically and theoretically. This talk focuses on a recent development in monopolistic setting of so-called real-time dynamic pricing (RTDP), which is aimed at reducing the computational complexity of dynamic pricing for large-scale implementation. RTDP only needs to solve a large-scale optimization once, which can be done offline, and it automatically adjusts the prices on the fly as demands are realized. To build an intuition, I will first describe a version of RTDP that has been studied in the context of standard Revenue Management (RM) problem. I will then discuss a recent development in the setting of RM with click models.
|