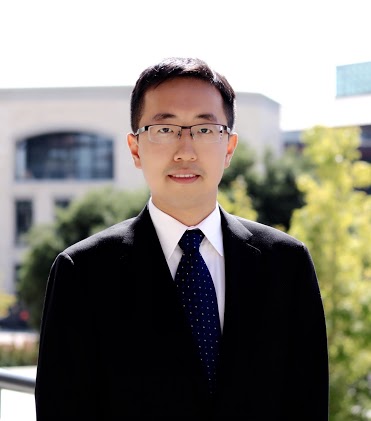
| Zeyu Zheng
加州伯克利大学工业工程与运筹系副教授
|

|
【主讲】Zeyu Zheng,加州伯克利大学工业工程与运筹系副教授
Zeyu Zheng is an Assistant Professor in the Department of Industrial Engineering & Operations Research (IEOR) at UC Berkeley. He received his Ph.D. in management science and engineering (2018), Ph.D. minor in statistics (2018), and MA in economics (2016), from Stanford University. Previously, he received a B.S. in mathematics (2012) from Peking University. His has done research in simulation, stochastic modeling, data-oriented decision making, and financial intermediation.
【Speaker】Zeyu Zheng, Assistant Professor, Department of Industrial Engineering & Operations Research (IEOR) at UC Berkeley
【Topic】Non-stationary Markov processes: Approximations, simulation, and decision-making【Time】Wednesday, June 5, 2019, 9:30
【Venue】Room 453, Weilun Building, Tsinghua SEM
【Organizer】Department of Management Science and Engineering
【Abstract】 In many Markov modeling contexts, the system under consideration exhibits strong time-of-day effects, day-of-week effects, or seasonality effects. In fact, most real-world systems that are modeled as Markov processes exhibit such non-stationarities. Nevertheless, the great majority of the academic literature focuses on modeling and theory for Markov processes with stationary transition probabilities, which describe systems that have no such time-of-day effects or seasonality. In this talk, we will describe an analytical approximation approach to handle non-stationary Markov models, anchored on traditional stationary analysis, that are available when the transition probabilities are slowly changing or rapidly changing over the time horizon in question. We will then discuss the use of Monte Carlo simulation to study such non-stationary processes, followed by implications on decision-making under non-stationarities.
|