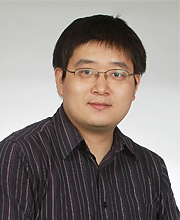
| Ning Cai
香港科技大学工业工程及决策分析学系副教授
|

|
【主讲】蔡宁,香港科技大学工业工程及决策分析学系副教授
【时间】2019年4月25日(周四)上午10:30am – 12:00pm
蔡宁是香港科技大学工业工程及决策分析学系的副教授。他是香港科技大学金融科技硕士项目(由工学院,理学院和商学院联合主办)的首任Academic Director,同时担任工业工程及决策分析学系金融工程及金融科技实验室的Director。蔡宁于2008年在哥伦比亚大学获得运筹学博士学位,并分别于2000年和2003年在北京大学数学科学学院获得概率统计学士和硕士学位。他的研究方向主要包括金融工程,金融科技,数据科学,应用概率以及金融和经济中的随机建模。他现在担任Operations Research, Operations Research Letters, Digital Finance 和 IMA Journal of Management Mathematics的associate editor以及Probability in the Engineering and Informational Sciences的editorial board member.
【Speaker】Ning Cai, Associate Professor, Department of Industrial Engineering and Decision Analytics, Hong Kong University of Science and Technology
【Topic】FinTech, Data Analysis, and Privacy Preservation
【Time】Tuesday, April 25, 2019, 10:30am – 12:00pm
【Venue】Room 401, Weilun Building, Tsinghua SEM
【Organizer】Department of Management Science and Engineering
【Abstract】 Many data are sensitive in areas such as finance, economics, political science, and life science. How to protect individual privacy when collecting and analyzing data has become even more significant and has raised growing public concerns in the age of big data because a huge amount of personal data are being generated and used almost freely every day. We propose an ER (encryption and recovery) algorithm that allows a central administration to do statistical inference based on the encrypted data, while still preserving each party's privacy even for a colluding majority in the presence of cyber attack. Theoretically, we essentially establish a general framework for privacy-preserving statistical inference, which can be viewed as the sensitive data based counterpart of traditional statistical inference assuming availability of the data. We demonstrate the applications of our algorithm to linear regression, logistic regression, maximum likelihood estimation, estimation of empirical distributions, and estimation of quantiles. Moreover, our algorithm can help to address another practically significant issue -- privacy preservation for distributed statistical inference when data are allocated to different parties who are unwilling to share their own data with others. Our algorithm is a promising “technology” that can be applied to overcome the difficulties of data analysis with privacy preservation not only in financial industry but also in other areas concerning people's privacy rights in the era of big data.
|