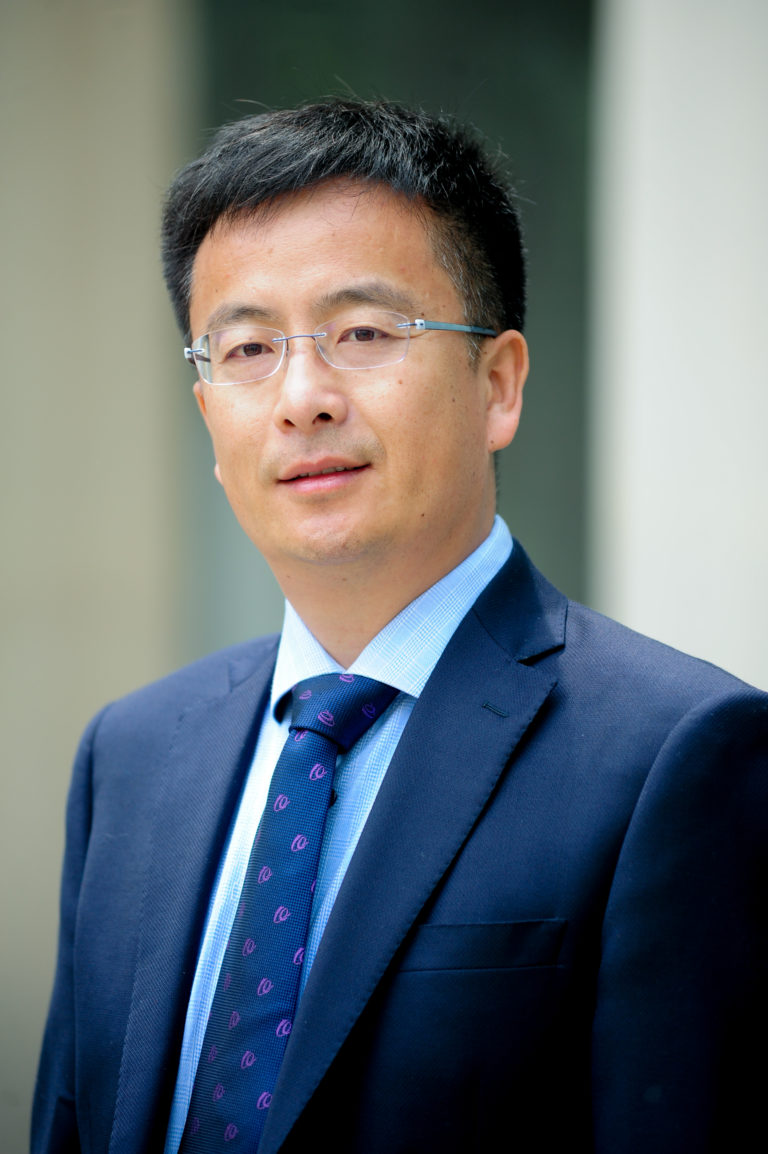
Prof. Max Zuo-Jun Shen
University of California at Berkeley
Talk:
Decision Making with Limited Information
Abstract:
I will discuss two recent papers, both of which have something to do with decision making with limited information. In the first half of the talk, I will present a dynamic assortment optimization. Given the limited store capacity, a retailer must decide which products to offer to customers to maximize the expected profit. We assume that each customer chooses the product that maximizes her utility and consider the multinomial logit choice model to represent demand. The retailer, however, does not know the demand for each product. She can learn the demand distribution by offering different product assortments, observing resulting purchases, and inferring the demand distribution from past sales and assortment decisions. We present an adaptive algorithm for joint parameter estimation and assortment optimization. To evaluate our proposed policy, we define a benchmark profit as the maximum expected profit that the retailer can earn if she knows the demand distribution in advance. We show that the running average expected profit generated by our algorithm converges to the benchmark profit and establish its convergence rate. Numerical experiments based on sales data from an online retailer indicate that our policy performs well. In the second half of the talk, I will discuss an infrastructure design problem for electric vehicles (EVs). A key obstacle to infrastructure planning for EVs comes from the long recharging time that makes it difficult to "refuel" the vehicle in the middle of a long trip. To address this problem, some have advocated that an EV infrastructure network should include swapping stations, at which EVs may exchange their empty batteries for full ones in the middle of long trips, in addition to charging adaptors at homes, work places and shopping malls. This solution allows EVs to be effectively "refueled" in only 1-2 minutes instead of hours. Since the adoption rate of electric vehicles, and thus demand for swapping service, is still highly uncertain, we model the swapping station network design problem within a robust optimization framework. The objective is to minimize the cost of servicing customers while maintaining a certain service requirements. We demonstrate how to tightly bound this integrated network design problem by a mixed-integer second-order cone programming problem. Finally, we discuss some computational results using data from the San Francisco Bay Area freeway network.
Biography:
Zuo-Jun (Max) Shen received his Ph.D. from Northwestern University. He has been active in the following research areas: integrated supply chain design and management, market mechanism design, applied optimization, and decision making with limited information. He is currently on the editorial/advisory board for several leading journals. He received the CAREER award from National Science Foundation in 2003.