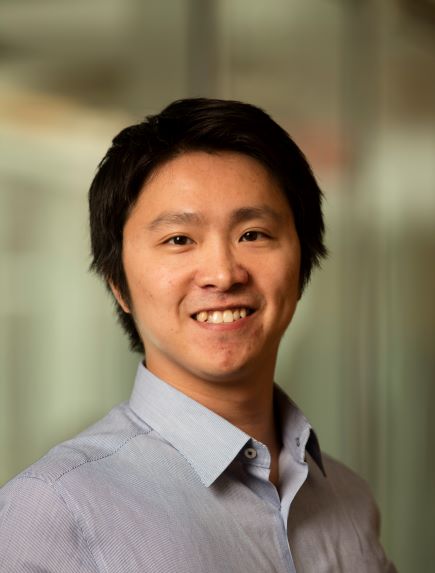
Prof. Henry Lam
Assistant Professor, University of Michigan
Talk:
Pooling Queues with Work-Averse Servers
Abstract:
We discuss a statistical framework to integrate data into optimization under uncertain constraints, via the use of suitably constructed robust optimization (RO) that provides inner approximation to a benchmark chance-constrained problem. The framework is based on learning a prediction set with geometries compatible with established RO tools and validating the set to achieve finite-sample statistical guarantees on feasibility. We present and compare the performances and sample size requirements of our method with previous approaches, and demonstrate how our framework provides a platform to integrate machine learning tools into uncertain optimization driven by convoluted or high-dimensional data.
*This is joint work with Jeff Hong and Zhiyuan Huang
Biography:
Henry Lam is currently an Assistant Professor in the Department of Industrial & Operations Engineering at the University of Michigan, Ann Arbor. He is interested in building robust and statistically principled methodologies for Monte Carlo simulation, risk analysis, and stochastic and simulation-based optimization. Henry Lam received his Ph.D. degree in statistics at Harvard University in 2011.