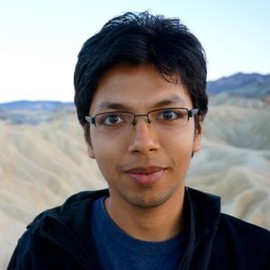
Prof. Siddhartha Banerjee
Assistant Professor, Cornell University
Talk:
Optimization and Market Design in Shared Vehicle Systems
Abstract:
Shared vehicle systems, such as those for bike-sharing (e.g., Citi Bike in NYC, Velib in Paris), car-sharing (e.g., car2go, Zipcar) and ridesharing (Uber, Lyft, etc.) are fast becoming essential components of the urban transit infrastructure. The technology behind these platforms enable fine-grained monitoring and control; however, owing to their on-demand nature and network externalities, optimizing such systems is challenging. I will present two recent projects in which we have developed new tools for controlling such systems. First, I will describe a unifying framework for data-driven pricing and optimization in shared vehicle systems, which provides efficient algorithms with rigorous guarantees under a variety of controls (pricing, empty-vehicle repositioning, demand redirection) and for a wide class of objective functions (welfare, revenue, multi-objective problems such as Ramsey pricing). Next, using the particular example of dynamic pricing in ride-sharing platforms, I will discuss how market mechanisms can help us to go beyond data-driven optimization in such systems, by allowing the platform to realize optimal performance with limited knowledge of system parameters; in particular, I will show how dynamic pricing allows the platform to realize optimal performance with limited knowledge of system parameters. Based on joint work with Ramesh Johari and Carlos Riquelme at Stanford, Daniel Freund and Thodoris Lykouris at Cornell, and the data science team at Lyft.
Biography:
Siddhartha Banerjee is an assistant professor in the School of Operations Research and Information Engineering (ORIE) at Cornell, where he works on stochastic modeling, and the design of algorithms and incentives for large-scale systems.His research focusses on settings where a large number of agents interact, either via an explicit network (e.g., in communications/social networks), or via implicit networks arising due to an algorithm (e.g., in recommender systems) or an incentive mechanism (e.g., in matching markets and social-computing platforms). He is most excited by problems where theory provides sharp qualitative insights for the design of real platforms, and where resulting algorithms and mechanisms can be implemented in practice.