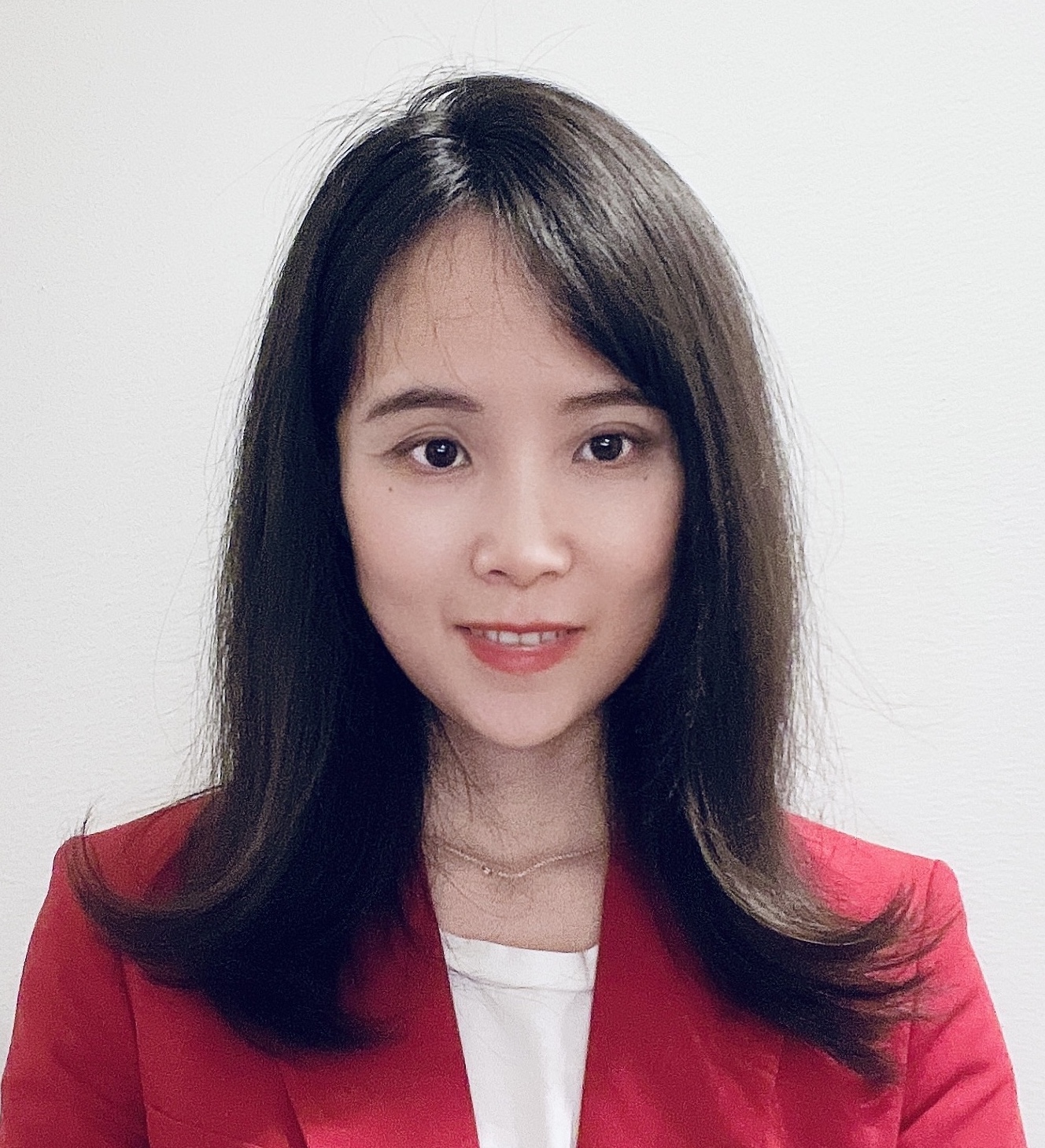
Prof. Yao Xie
Georgia Institute of Technology
Talk:
Flow-based Distributionally Robust Optimization
Abstract:
We present a computationally efficient framework, called 𝙵𝚕𝚘𝚠𝙳𝚁𝙾, for solving flow-based distributionally robust optimization (DRO) problems with Wasserstein uncertainty sets while aiming to find continuous worst-case distribution (also called the Least Favorable Distribution, LFD) and sample from it. The requirement for LFD to be continuous is so that the algorithm can be scalable to problems with larger sample sizes and achieve better generalization capability for the induced robust algorithms. To tackle the computationally challenging infinitely dimensional optimization problem, we leverage flow-based models and continuous-time invertible transport maps between the data distribution and the target distribution and develop a Wasserstein proximal gradient flow type algorithm. In theory, we establish the equivalence of the solution by optimal transport map to the original formulation, as well as the dual form of the problem through Wasserstein calculus and Brenier theorem. In practice, we parameterize the transport maps by a sequence of neural networks progressively trained in blocks by gradient descent. We demonstrate its usage in adversarial learning, distributionally robust hypothesis testing, and a new mechanism for data-driven distribution perturbation differential privacy, where the proposed method gives strong empirical performance on high-dimensional real data. Joint work with Chen Xu and Jonghyeok Lee at the Georgia Institute of Technology and Xiuyuan Cheng at Duke University.
Biography:
Yao Xie is the Coca-Cola Foundation Chair, Professor at Georgia Institute of Technology in the H. Milton Stewart School of Industrial and Systems Engineering, and Associate Director of the Machine Learning Center. From September 2017 until May 2023, she was the Harold R. and Mary Anne Nash Early Career Professor. She received her Ph.D. in Electrical Engineering (minor in Mathematics) from Stanford University in 2012 and was a Research Scientist at Duke University. Her research lies at the intersection of statistics, machine learning, and optimization in providing theoretical guarantees and developing computationally efficient and statistically powerful methods for problems motivated by real-world applications. She received the National Science Foundation (NSF) CAREER Award in 2017, the INFORMS Wagner Prize Finalist in 2021, and the INFORMS Gaver Early Career Award for Excellence in Operations Research in 2022. She is currently an Associate Editor for IEEE Transactions on Information Theory, Journal of the American Statistical Association-Theory and Methods, Operations Research, Sequential Analysis: Design Methods and Applications, INFORMS Journal on Data Science, and an Area Chair of NeurIPS, ICML, and ICLR.