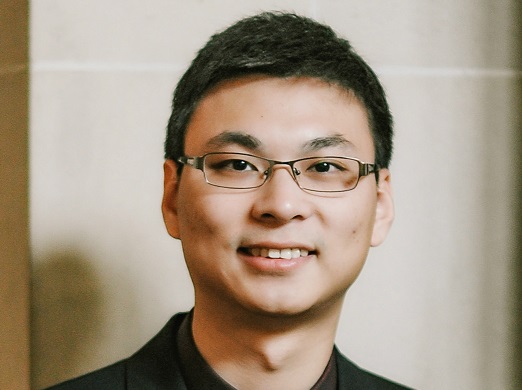
【主讲】Long He,新加坡国立大学商学院助理教授
【主题】用于应急响应的空间数据驱动无人机布局
【时间】2020年4月29日(周三)上午 10:00am
【地点】线上:zoom软件,会议ID: 662 5582 0994
【语言】英文
【主办】管理科学与工程系
【简历】Long He教授简历
Long He is an assistant professor in Department of Analytics & Operations (DAO) at NUS Business School, National University of Singapore. He received his Ph.D. in Operations Research from the University of California, Berkeley, and his B.Eng. in Logistics Management and Engineering from HKUST. His current research involves using data-related approaches to address problems in smart city operations (e.g., vehicle sharing, last-mile delivery) and supply chain management. He has received MSOM best paper award and INFORMS TSL best paper award.
【Speaker】Long He, Assistant Professor, NUS Business School, National University of Singapore.
【Topic】Spatial-Data-Driven Drone Placement for Emergency Response
【Time】Wednesday, April 29, 2020, 10:00am
【Venue】Online: zoom, Meeting ID: 662 5582 0994
【Language】English
【Organizer】Department of Management Science and Engineering
【Abstract】
With recent technological advancements, there is a growing interest in deploying unmanned aerial vehicles (UAVs), commonly known as drones, in providing assistance, delivering supplies, and rescue operations during emergency situations. To ensure quick response to a future emergency, it is crucial to strategically deploy the drones. In this work, we consider drone placement problem as a continuous p-median facility location problem under the spatial uncertainty associated with future emergency locations. We propose a spatial-data-driven model to deal with the key challenges, namely the continuous region of potential emergencies, sparse historical incidents, and non-stationarity of spatial distribution. Specifically, our solution approach involves three steps --- First, leveraging the historical data, we partition the entire region into several disjoint convex subregions; Using the empirical spatial distribution of historical occurrences as a reference, we then construct a spatial ambiguity set about the future spatial distribution such that the future spatial distribution lies within a certain Wasserstein distance of the reference distribution; Finally, under l1 Wasserstein distance, we derive the robust p-median location problem as a mixed-integer second-order cone program based on the vertices of those subregions. In a case study on Arizona wildfire fighting, our numerical results suggest that our model produces robust drone base station locations that hedge against the spatial uncertainty, and outperformed the sample average approximation approach in terms of quick response and fair accessibility.